ABSTRACT
We propose a method for online sensor fa ult detection that is based on the evolving Strong Tracking Filter (STCKF). The cubature rule is used to estimate states to improve the accuracy of making estimates in a nonlinear case. A residual is the difference in value between an estimated value and the true value. A residual will be regarded as a signal that includes fault information.
The threshold is set at a reasonable level, and will be compared with residuals to determine whether or not the sensor is faulty. The proposed method requires only a nominal plant model and uses STCKF to estimate the original state vector. The effectiveness of the algorithm is verified by simulation on a drum-boiler model.
PROBLEM FORMULATION
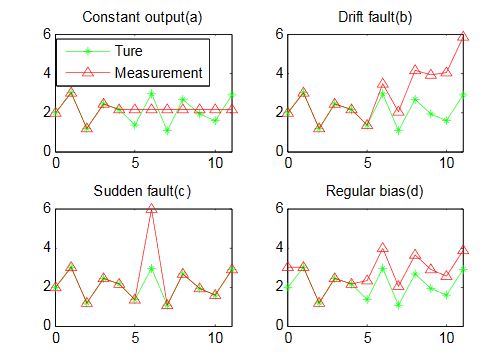
Figure 1. Common sensor fault types: (a) Constant output fault; (b) Drift fault
We consider a process that is actuator fault free and system fault free. The goal is to detect sensor failures. Sensor faults can be summarized as falling under four categories, as illustrated in Figure 1. We consider that only one type of fault at a time occurs on a sensor.
ALGORITHM FOR FAULT DETECTION
In this section a cubature rule is used in the process of estimating the stat e of a strong STF, and in generating the residual to judge whether or not a fault has occurred. If a fault has occurred, an alarm signal will be generated. We designed a series of filters to generate residuals for detecting sensor faults.
EXPERIMENTAL ANALYSIS
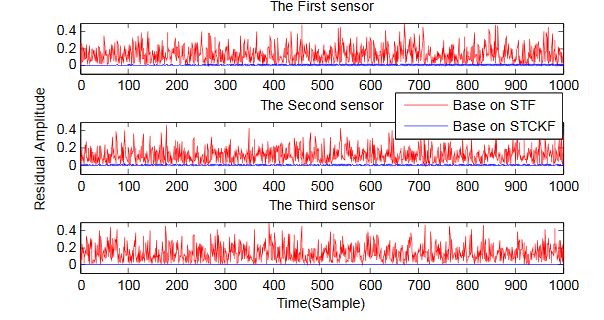
Figure 2. The sensor outputs residual in a fault- free case obtained by STCKF, UKF, and STF
Under fault-free conditions, the absolute value of three residuals, obtained through the STCKF, UKF, and STF, respectively, are compared in Figure 2. Comparing the residuals obtained through the three different algorithms, it is evident that STCKF has the highest accuracy. Residuals obtained by STCKF under a fault-free condition are very smooth. The STCKF can reduce rates of false alarms and missed alarms in the fault detection process.
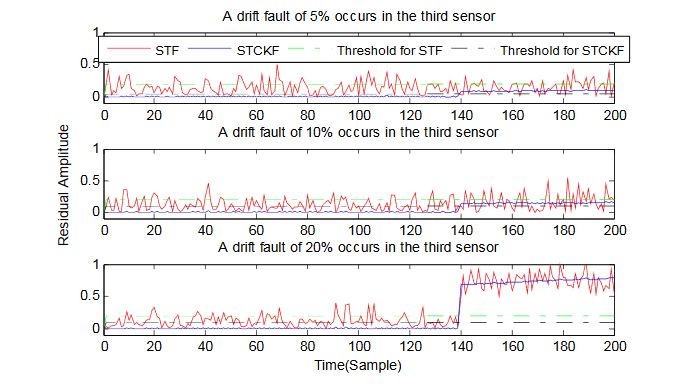
Figure 10. A drift fault occurs only in the third sensor, based on STCKF, UKF, and STF, at t ≥ 140
The STF method produces larger errors than the other methods in the prediction process for strong nonlinear systems, and this has a negative effect on fault detection. In the case of small regular bias faults and drift faults, as shown in the first picture in Figure 10, the STF method cannot even produce effective residuals. Because the residual value obtained by STCKF is smooth under fault-free conditions and exhibits obvious changes when the sensors come across failure, we can use a simple method to set a fixed threshold for fault detection and achieve good implementation.
CONCLUSIONS
In this paper, a new approach to online sensor fault detection was proposed. Moreover, this approach offers the advantage of accurate and simple calculations. With the aim of obtaining more accurate estimates, which can make the residuals smooth, thereby reducing the rates of missed alarms and false alarms, a cubature rule was introduced in the STF. For strong nonlinear systems, the proposed algorithm offers a great improvement in accuracy.
It generated smooth residuals in fault-free cases, which made the detection of faults accurate. To evaluate the proposed approach, it was applied to a nonlinear model of a drum-boiler. The results of the simulation confirmed that this method is more efficient at online sensor fault detection. The case of the faults of different, multiple interrelated sensors will be the focus of the next stage of research.
Source: Capital Normal University
Authors: Lijuan Wang | Lifeng Wu | Yong Guan | Guohui Wang