ABSTRACT
Following the remarkable developments in computing and wireless communication technologies, there has been a rapid proliferation of mobile and embedded computing systems and applications that are becoming ubiquitous in all aspects of modern life, including the enterprise world, the entertainment world as well as in common household appliances. However, designers of new generations of these systems have to address a twofold demand for increasing application functionality as well as a reduction in device footprints.
This dissertation proposes a design principle that can allow mobile computing applications to transcend the limitations on the end-devices, by splitting up the functionality of the application end points between the low-footprint wireless end-nodes and shared fixed nodes inside the network. It presents two instantiations of this idea in different types of wireless networks, identifying and solving some of the key challenges faced when applying the proposed principle.
In the first part, the focus is on wireless access networks, where shared processing resources within the network can be used to support high-end applications on thin handheld clients. A major challenge here is to determine how to schedule the wireless communication and computing resources together to support a large set of clients. The second part of the dissertation demonstrates how the same design principle can be applied to wireless sensor networks to enable the use of simple, ultra-low-power sensor nodes.
The resource limitations on the sensor nodes make it challenging to ensure the reliability of the sensor data, and a novel solution is proposed that leverages the correlation properties of the data to do so. The experimental results presented demonstrate the viability of the proposed architectural principle in systems that vary markedly in terms of application goals and device capabilities. It is shown that the technique proposed in the first part can achieve very efficient scheduling performance with minimal processing overheads.
This enables the expansion of the application functionality without increasing the footprint of the end-devices. Similarly, in sensor networks, the error correction method demonstrated the feasibility of achieving the primary functionality, i.e., reliable data collection even when the footprints of the sensor nodes are reduced too far to implement traditional reliability measures. The techniques described will facilitate the adoption of infrastructure-based approach to system design, leading to high-end applications using low-footprint devices.
ENABLING RICH MOBILE APPLICATIONS: JOINT COMPUTATION AND COMMUNICATION SCHEDULING
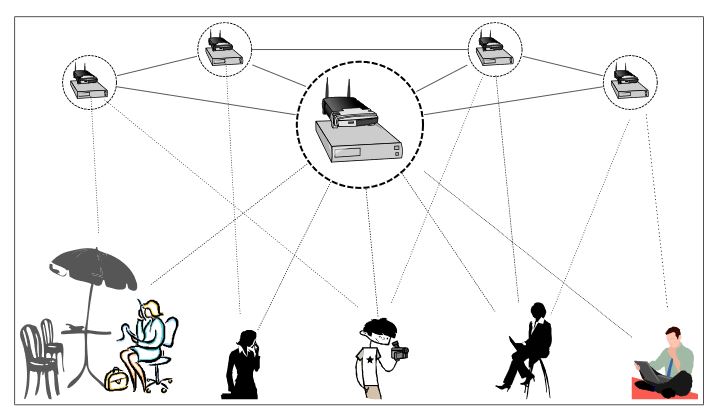
Figure 2.1: Network of enhanced wireless LAN with additional processing elements co-located with the access points
We envision that the ubiquitous availability of this computing infrastructure can be leveraged to support the heavy computational tasks for the mobile applications, and use a thin-client design for the low-footprint part of the end nodes. In our work, we consider one implementation for this architecture where the computing support is built into the wireless access points, which could provide both computation and communication support for mobile applications, as illustrated in Figure 2.1.
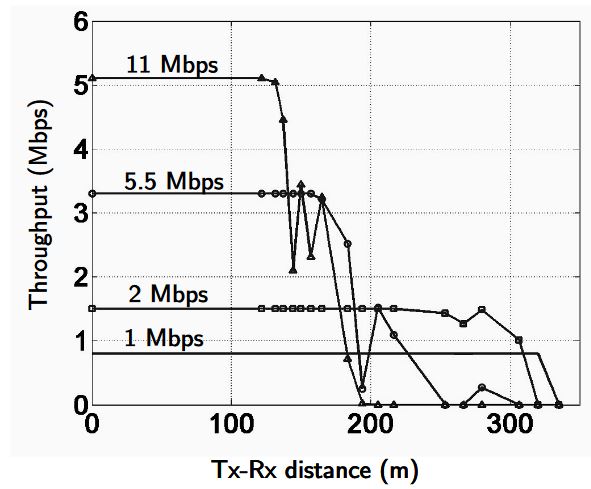
Figure 2.5: Measured Throughput vs. Range of 802.11b for outdoor transmission between a single pair of nodes
The numbers in the table are based on throughput vs. range characteristics (See Fig. 2.5), as measured on CalRadio, a 802.11-based experimental platform with a software-programmable MAC which was developed in our group [JSP07]. The interference model that the scheduler operates on is I = 2R (I =interference range, R =transmission range), as described in Section 2.3.
MODEL BASED TECHNIQUES FOR DATA RELIABILITY IN WIRELESS SENSOR NETWORKS
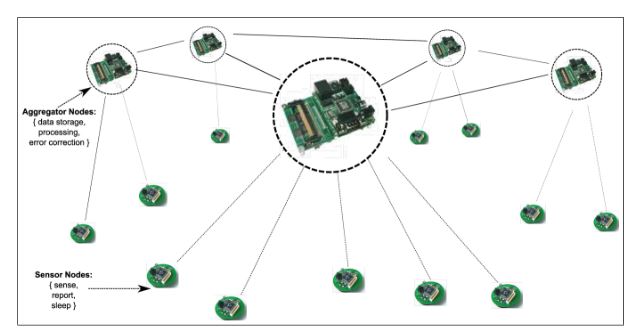
Figure 3.1: Network of sensor nodes and clusterheads
A second type of nodes, called aggregator nodes representing the shared fixed node in the same figure. These are equipped with substantial data processing capabilities and can connect to one or more sensor nodes. The resulting architecture is shown in Fig. 3.1, with two types of customized sensor nodes available today representing the sensor and aggregator nodes respectively. In practice, the aggregator nodes can be made with generic off-the-shelf processors, while the sensor nodes are expected to be even smaller and simpler.
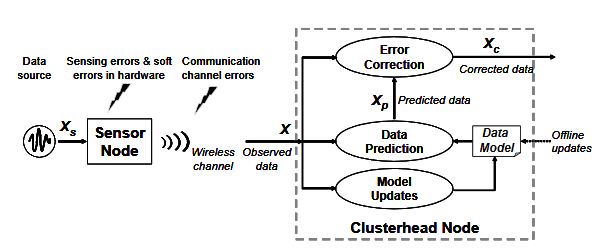
Figure 3.2: Overall scheme for model-based error correction
There are two main parts in this method: data modeling and correction. The first step is the construction of the data model by analysis of the properties of the source data. Certain statistical properties will be generally applicable to the type of application, and may be identified by offline analysis, e.g., knowing that correlation time is on the order of hours rather than milliseconds. Other properties will need to be identified for each sensor and need run time tuning of the data model. Once a model is identified, it is used during normal operation of the network to detect and correct errors, as illustrated in Fig. 3.2.
CONCLUSIONS AND FUTURE DIRECTIONS
This chapter concludes the dissertation with a summary of our principal contributions and some thoughts about the implications of this research for future generations of systems. The underlying idea of this work has been the architectural principle of splitting the functionality of the end nodes, as presented in Figure 1.3 in Chapter 1. In subsequent chapters, we looked at two different types of wireless networks where this idea was realized to satisfy different goals specific to each system.
In Chapter 2, we presented an architecture for a wireless network where the wireless access points are enhanced with embedded processing resources, which enables them to support some of the application-specific processing for the client nodes. For this application, we addressed the problem of sharing the processing resources in the network among end nodes, by a designing a set of heuristic solutions for joint scheduling of computation and communication. We showed that these heuristics allow efficient usage of these shared resources in such a network architecture.
As a result, this architecture can be successfully used to enable rich applications with enhanced functionality, while continuing to use low-footprint devices as clients. In Chapter 3, we focused on wireless sensor networks, and showed how a hierarchical network architecture can allow the use of ultra low-footprint sensor nodes, and still remain capable of performing reliable data collection. To achieve this, we presented a method for correcting errors in sensor data that uses patterns and properties of the sensor data itself.
Our method moved the processing overheads of error correction out of the thin sensor nodes and into the more powerful cluster-head nodes, and in the process effectively allowed the sensor nodes to be made smaller and thinner. Though the types of systems covered in the last two chapters differed drastically in terms of goals and functionality, they can both be considered to be instantiations of the same architectural principle that was presented in Chapter 1.
In both cases, the proposed architectural idea involved splitting what was formerly the functionality of the end nodes into two different parts. In the first instance, the resulting architecture allows the extension of the functionality of mobile applications even as the footprints of the end nodes continued to remain low. In the second case, the use of the same principle enables significant reduction in the footprints of the sensor nodes without sacrificing the primary functionality of the system, which is reliable data collection.
The two examples illustrate the ability of the proposed architectural principle to solve some of the technical challenges of next generations of wireless computing systems. As discussed in Chapter 1, there has been a convergence of computing and communication functionalities in the present generation of wireless networks, and future generations of these systems have to address the twin goals of enhanced functionality and reduced footprints.
For any given generation of technology, any design involves a trade-off between these two goals. What we have demonstrated in this dissertation is that another approach, consisting of an architectural redesign, can transcend this trade-off in a way that is not feasible without breaking the current architecture. The architectural change comes with its own effects on the application design, and in the previous chapters we demonstrated how some of these challenges can be addressed in the context of two types of systems with widely disparate requirements and constraints.
Source: University of California
Author: Shoubhik Mukhopadhyay
>> More Wireless Sensor Networks Projects Abstract for Engineering Students
>> More Wireless Embedded Projects for Engineering Students