ABSTRACT
An intelligent control of photovoltaics is necessary to ensure fast response and high efficiency under different weather conditions. This is often arduous to accomplish using traditional linear controllers, as photovoltaic systems are nonlinear and contain several uncertainties. Based on the analysis of the existing literature of Maximum Power Point Tracking (MPPT) techniques, a high performance neuro-fuzzy indirect wavelet-based adaptive MPPT control is developed in this work.
The proposed controller combines the reasoning capability of fuzzy logic, the learning capability of neural networks and the localization properties of wavelets. In the proposed system, the Hermite Wavelet-embedded Neural Fuzzy (HWNF)-based gradient estimator is adopted to estimate the gradient term and makes the controller indirect. The performance of the proposed controller is compared with different conventional and intelligent MPPT control techniques. MATLAB results show the superiority over other existing techniques in terms of fast response, power quality and efficiency.
PHOTOVOLTAIC ENERGY SYSTEM
The PV system generates electricity from solar irradiance. The PV structural unit contains solar cells. The entire layout of the PV system interconnecting with the load is shown in Figure1. The system is comprised of a DC-DC boost converter.
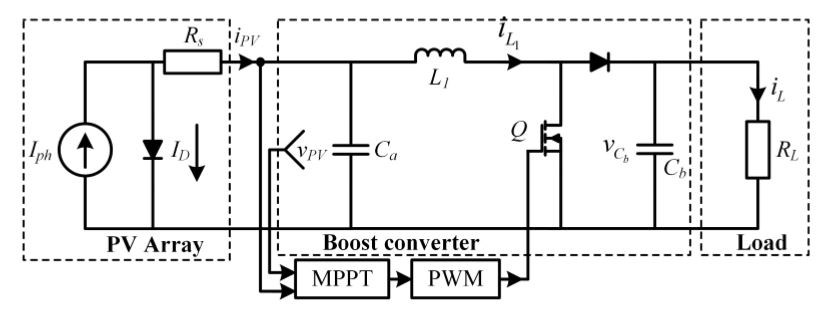
Figure 1. Photovoltaic energy system
Dc-dc Boost Converter
The schematic diagram of the DC-DC boost converter is shown in Figure1. Basically, this converter is required to track the PVMPP by adjusting its duty cycle D between [0,1]. A Pulse Width Modulation (PWM) generator is used to generate the appropriate pulse signal to MOSFETQ according to the given duty cycle. The DC-DC boost converter is characterized by its non-linearity.
PROPOSED ADAPTIVE NEURAL FUZZY CONTROL SYSTEM
The indirect adaptive neural fuzzy control system is used to control PV output power. Initially, the Hermite Wavelet-based Adaptive Neural Fuzzy Controller (HWANFC) is adopted as the MPP tracker for the PV system. In the proposed system, the HWNF-based gradient estimator is adopted to estimate the gradient term and makes the controller indirect. The proposed intelligent control system is shown in Figure 2. An HWANFC is used as an MPP tracker.
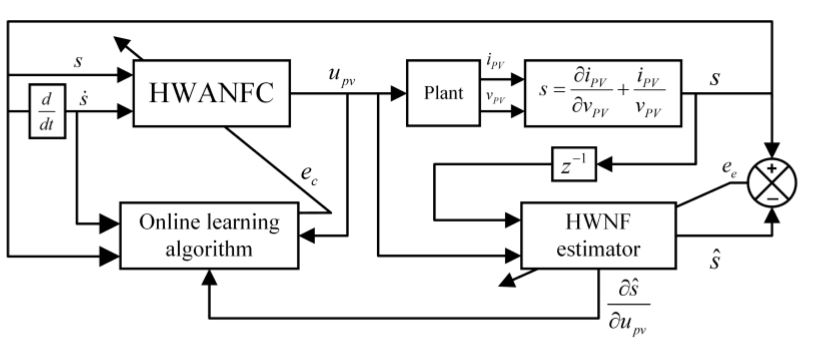
Figure 2. Adaptive Hermite Wavelet-based Adaptive Neural Fuzzy Controller (HWANFC) control system
Figure 3 depicts the HWANFC structure, which is a five-layer feed forward connectionist network.
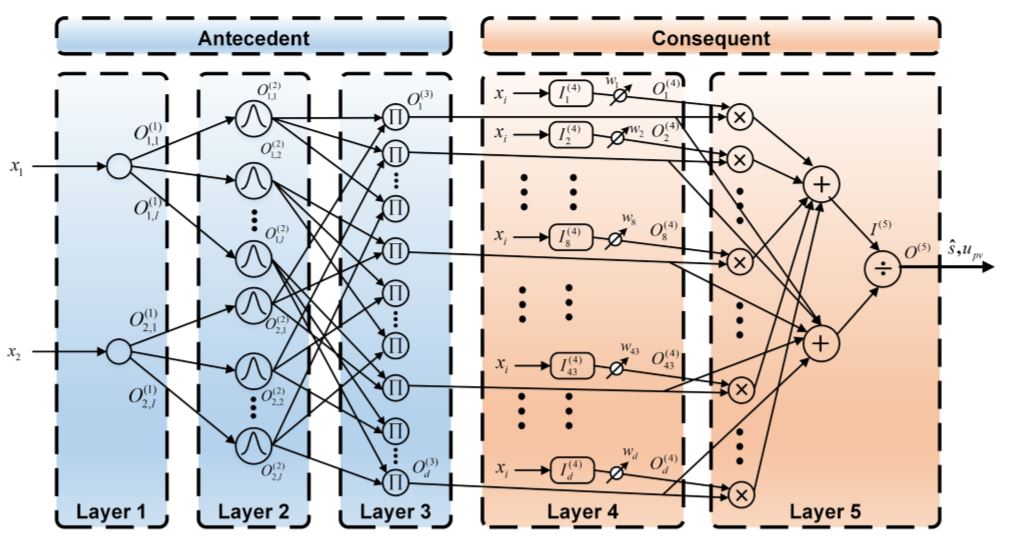
Figure 3. Structure of the proposed HWANFC and HWNF-based gradient estimator
RESULTS AND DISCUSSION
The PV array containing 66 series with 13 parallel strings is constructed via the Sim power System toolbox. The overall power rating of the PV array is 261 kW. In order to prove the effectiveness of the proposed control algorithm, a traditional Fuzzy Logic Controller (FLC) based on the IC algorithm, a PID controller based on IC and the P&O algorithm are also used to track MPP. In this research, the Defense Housing Authority (DHA), Islamabad, Pakistan, is taken as a case study. The minute basis irradiance (W/m2) and ambient temperature (◦C) levels are recorded by the Pakistan Meteorological Department (PMD) for a complete summer day, as shown in Figure 4.
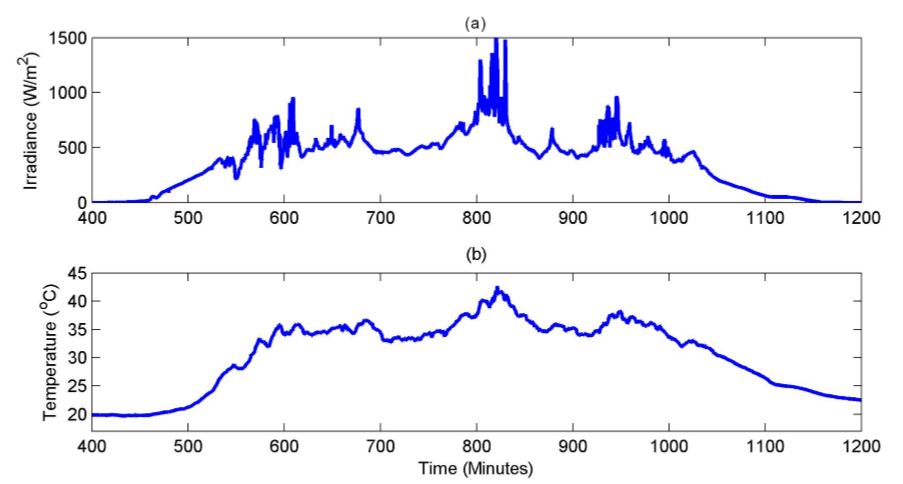
Figure 4. Atmospheric conditions: (a) solar irradiance; (b) ambient temperature
The comparison between error terms acquired by the controlled plant and the HWNFGE generated errors is illustrated in Figure 6. From the figure, it is clearly observed that the HWNFGE accurately identifies the network and calculates the error term efficiently. However, at large values of s (due to the rapid change in irradiance level), the value of $s is slightly less than the experimental error.
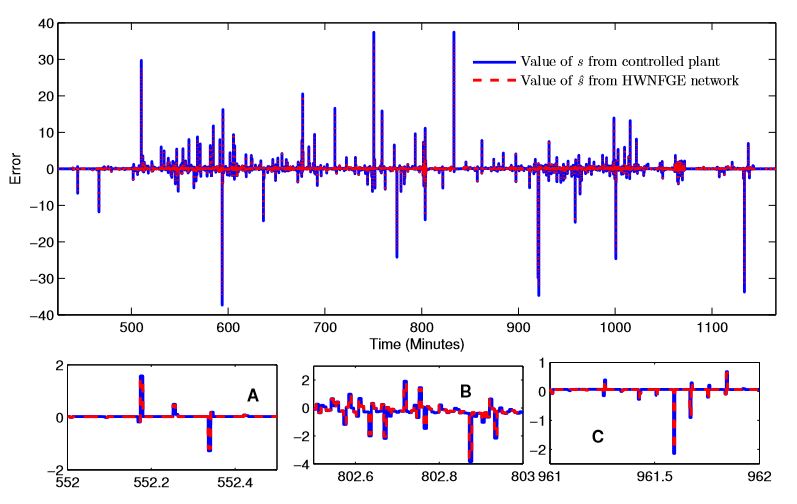
Figure 6. Comparison between experimental error s and HWNFGE generated errors (A) zoomed plot at t = 552–552.5 min (B) zoomed plot at t = 802.5–803 min; (C) zoomed plot at t = 961–962 min.
CONCLUSIONS
In this work, an intelligent wavelet-based neuro-fuzzy indirect method with high adaptive capability is designed for the MPPT of a PV system. A five-layer NFC is adopted as the process feedback controller. The proposed control is initialized from the traditional fuzzy control by means of expert knowledge, which decreases the weight of the lengthy pre-learning. With a derived learning scheme, the parameters are updated in the proposed structure adaptively by observing and adjusting the tracking error.
A neural network is developed to provide the HWANFC with the gradient information. The Hermite wavelets are integrated to improve the performance of the proposed controller. Various simulation results and comparison indexes have shown that the HWANFC can track the MPP quickly with high robustness to the parameter variations and external load disturbances and out-performs compared with the traditional MPPT techniques.
Source: Chongqing University
Authors: Syed Zulqadar Hassan | Hui Li | Tariq Kamal | Ug ur Arifog lu | Sidra Mumtaz | Laiq Khan
>> 200+ Matlab Projects based on Control System for Final Year Students
>> For More Matlab Projects on Embedded Systems for Final Year Students
>> More Neural Networks based Final Year Matlab Projects for Engineering Students